Are we confident enough to make data-informed decisions?
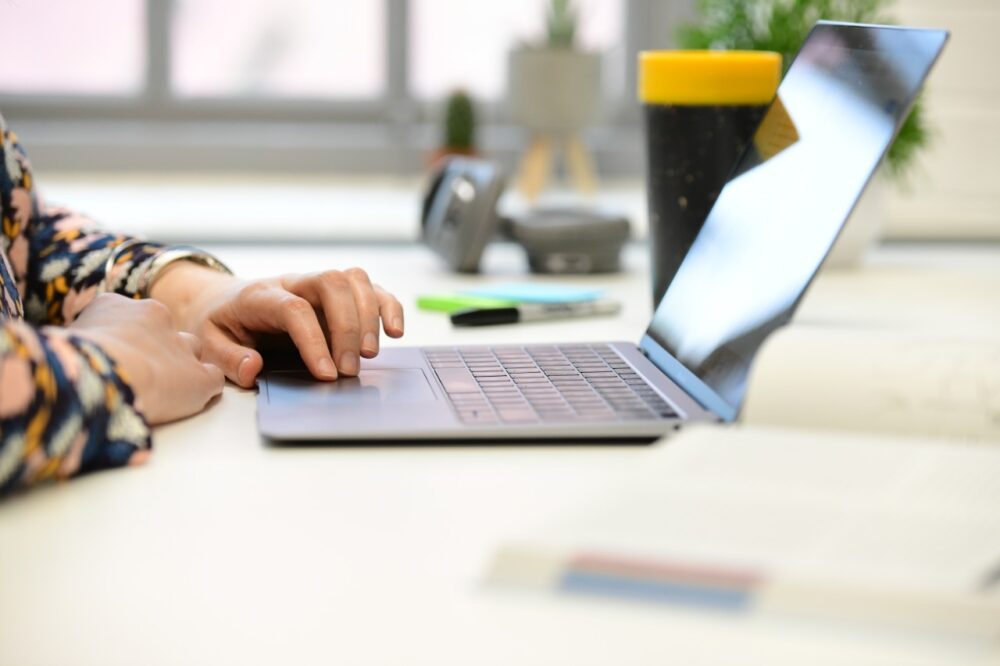
The real barrier seems to be confidence: in understanding, evaluating, and applying data to decision making
There’s lots of talk about the need to make data-informed decisions in government. From my experience working with different types of data across central and local government, the biggest challenges aren’t primarily technical, policy-related, or even about data quality – though all of these are challenges. The real barrier seems to be confidence: in understanding, evaluating, and applying data to decision making.
The path of least risk
It’s almost always considered more risky to share data than not to. Despite cases where not sharing information has led to bad outcomes, some teams continue to be nervous. Often, this leads to more talking about the need to share data than the practicalities of how to do it in a way everyone is comfortable with.
GDPR was a huge advance in how we treat personal information. However, it also brought with it a potentially disproportionate nervousness, with people overestimating the size and probability of risk. I’ve seen many examples of organisations that have a data privacy person or people to whom others outsource their confidence. This a big professional burden and an even bigger bottleneck to progress.
The 7th Calidcot principle says that the duty to share information can be as important as the duty to protect it. If we’re serious about making more data-informed decisions, we need more people to be confident about how to work with and share data safely.
How rigorous is rigorous enough?
Biomedical sciences have probably the highest level of research rigour. The stakes are high, and the difference between statistical and clinical significance can mean a treatment brought to market or not.
Another barrier to better data-informed decision making in government is the feeling that all research needs to be of a similar standard to be valid. There’s often a sense that if something’s not collected “properly” it’s not useful. Instead, we should be considering what’s proportional to the decision at hand and evidencing the reasons for the decisions made more transparently.
There are some very real risks when building a digital product. Information security is always the highest priority and treated very carefully. But there are other decisions where risks are smaller and less likely. In these cases, data-informed decisions can still be made but a less rigorous methodology can be applied.
For example, I did some work on improving the efficiency of a SQL view that was a data source for a Power Bi dashboard. It was a well used report, so there wasn’t zero risk but it wasn’t so risky that I needed a rigorous method for collecting data on it.
My simple approach: I ran the old SQL query 5 times and took an average run time from those. I did the same for the new query and compared the two. This gave me an estimated time saving. I knew the report was typically used by senior stakeholders. In theory, I could have found an estimated cost of their time and multiplied that by the time saving.
It’s important to remember that there’s no perfect method for collecting data. Instead, we need to build confidence in making decisions based on what’s rigorous enough in any given circumstances.
Source of the data
Closely related to the above is the confidence to understand and evaluate the source of the data. Climate change reporting is a good example. When reporting on air temperature, one geographical area’s dataset might come from a local weather station in a remote location and others from infrared sensors.
At a high level, these air temperature values would be considered reasonably comparable, despite their different collection methods.
We need to be confident not only in the data itself but also in our ability to understand how it was collected and what the possible limitations of that are. This doesn’t mean discrediting any one source entirely but instead understanding it as fully as we can.
Data-informed and choice
There is sometimes a feeling that data-informed decision making is a step towards removing the human element of the process entirely, which makes people nervous.
Even when given the exact same information, not everyone will make the same decision. It takes confidence to accept that and realise it doesn’t mean the information wasn’t important in the first place. Data will inherently have biases, and as humans, we can adjust for those biases when necessary.
An example of this is the European Union’s Gender Directive. Despite data which showed gender having a significant influence on healthcare claims, insurers were prohibited from using gender as a factor when setting premiums for health insurance. Data will give us insight but it’s up to us to decide how to move forward.
Sharing the responsibility across disciplines
Confidence in working with data can vary a lot and is often siloed to specific teams or disciplines. People who don’t have conventional ‘data’ titles can feel separate from ‘data work’, creating bigger gaps between them and those that do.
So, for decision makers who don’t come from a data-centric background, how easy is it to make confident, data- informed decisions?
Multidisciplinary teams work well when people have specialist experience but with a shared understanding of one another’s role. The more everyone can get involved with conversations about data and build their confidence, the more opportunity will arise to build, test and learn quickly and with less risk.
Normalising working with data
Building a culture of confident, data-informed decision making won’t happen overnight. But it starts with shared responsibility, practical understanding, and a willingness to question. The more we normalise working with data as part of everyday decision-making across roles, teams, and disciplines, the better positioned we are to deliver services that are not just efficient, but truly effective.